
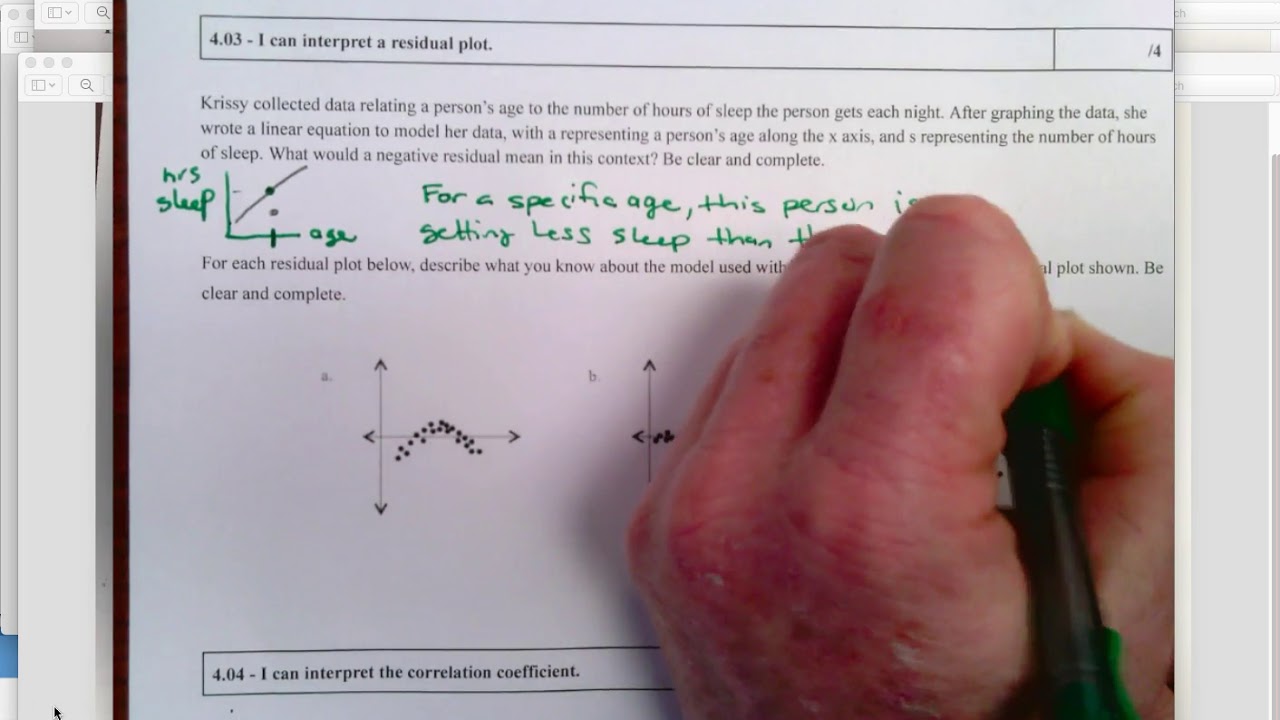
I used a fitted line plot because it really brings the math to life. So, a low p-value suggests that the slope is not zero, which in turn suggests that changes in the predictor variable are associated with changes in the response variable. If the fitted line was flat (a slope coefficient of zero), the expected value for weight would not change no matter how far up and down the line you go.
#Residual plots full#
The relationship is only valid within this data range, so we would not actually shift up or down the line by a full meter in this case. However, these heights are from middle-school aged girls and range from 1.3 m to 1.7 m. If you move left or right along the x-axis by an amount that represents a one meter change in height, the fitted line rises or falls by 106.5 kilograms. The blue fitted line graphically shows the same information. The coefficient indicates that for every additional meter in height you can expect weight to increase by an average of 106.5 kilograms. The equation shows that the coefficient for height in meters is 106.5 kilograms. The fitted line plot shows the same regression results graphically. I’ll illustrate this in the fitted line plot below, where I’ll use a person’s height to model their weight. The key to understanding the coefficients is to think of them as slopes, and they’re often called slope coefficients. This statistical control that regression provides is important because it isolates the role of one variable from all of the others in the model. Regression coefficients represent the mean change in the response variable for one unit of change in the predictor variable while holding other predictors in the model constant. Related: F-test of overall significance How Do I Interpret the Regression Coefficients for Linear Relationships? In the model above, we should consider removing East. Typically, you use the coefficient p-values to determine which terms to keep in the regression model. However, the p-value for East (0.092) is greater than the common alpha level of 0.05, which indicates that it is not statistically significant. In the output below, we can see that the predictor variables of South and North are significant because both of their p-values are 0.000. In other words, a predictor that has a low p-value is likely to be a meaningful addition to your model because changes in the predictor's value are related to changes in the response variable.Ĭonversely, a larger (insignificant) p-value suggests that changes in the predictor are not associated with changes in the response. A low p-value (< 0.05) indicates that you can reject the null hypothesis. The p-value for each term tests the null hypothesis that the coefficient is equal to zero (no effect). How Do I Interpret the P-Values in Linear Regression Analysis?
#Residual plots how to#
In this post, I’ll show you how to interpret the p-values and coefficients that appear in the output for linear regression analysis.
#Residual plots software#
After you use Minitab Statistical Software to fit a regression model, and verify the fit by checking the residual plots, you’ll want to interpret the results. Residuals for the package design example are given below.Regression analysis generates an equation to describe the statistical relationship between one or more predictor variables and the response variable.
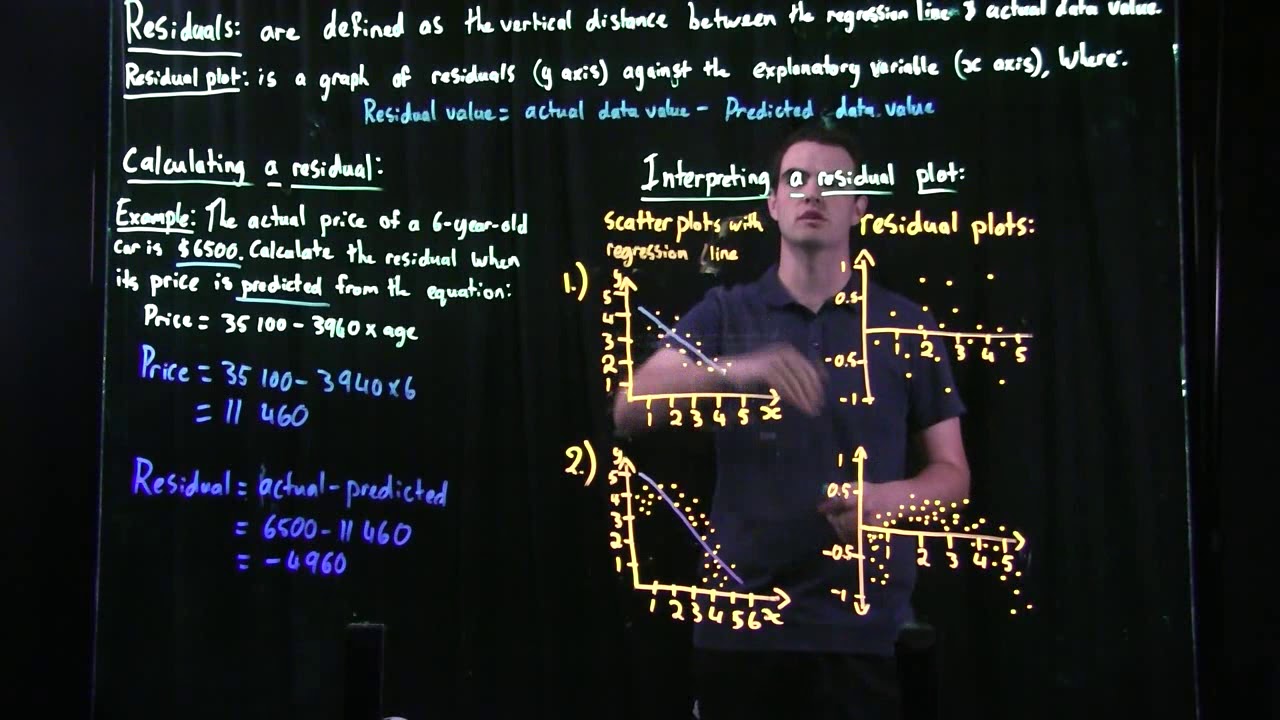
